Fill out the form to get more information about the USD bootcamp of your choice.
04.12.2024
Machine Learning vs. AI: Similarities and Differences
By The Fullstack Academy Team
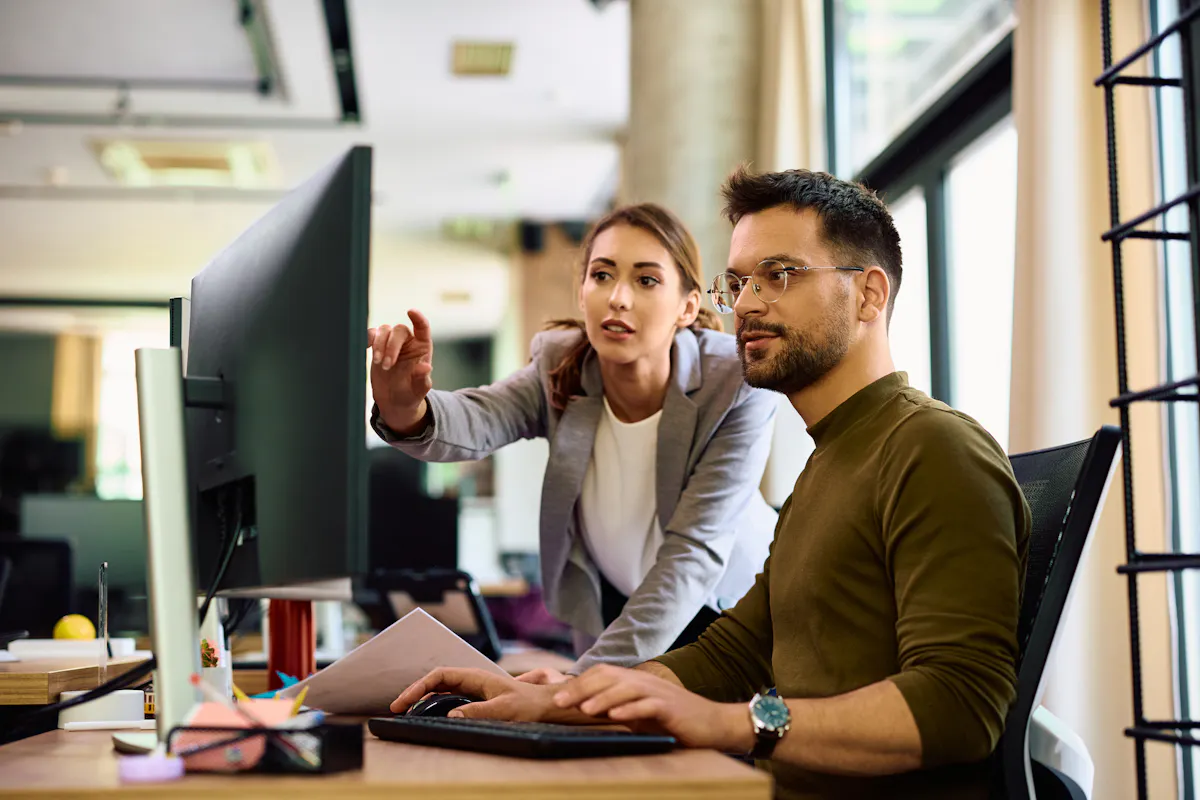
Machine Learning vs. AI: Similarities and Differences
Artificial intelligence (AI) and machine learning are transforming how business is done. Many industries, including retail and healthcare, are adopting AI and machine learning in their operations and business processes.
In retail, these technologies can improve businesses’ operations and help make customers happier. For example, they can analyze historical data to help predict what future customers will want to buy and when. In turn, retailers can make smarter choices about their inventory and prices.
In the healthcare industry, AI and machine learning can quickly analyze large volumes of patient data and image files. For doctors, this can mean uncovering hidden patterns in their patients’ data to help improve diagnoses and treatments.
Although these terms are often used interchangeably, AI and machine learning are not the same thing. In fact, machine learning is a subset of AI. However, when considering machine learning vs. AI from a market perspective, it’s clear they are similar in an important way: as they both are projected to experience strong growth over the next decade. Spherical Insights predicts the global machine learning market will grow from about $15 billion in 2021 to $302 billion in 2030. Statista data shows an expected AI market expansion of $200 billion in 2023 to $2 trillion by 2030.
This projected growth means numerous professional opportunities are likely to be available to those interested in pursuing AI and machine learning careers. Individuals with specialized training in AI and machine learning may have an edge. Understanding the differences between machine learning vs. AI is important for aspiring professionals.
What Is AI?
The concept of artificial intelligence was introduced in the 1950s when Alan Turing used his “Turing test” to assess a machine’s effectiveness in imitating human conversation. Participants in the test were asked whether they thought a response was from a machine or a human.
The definition has evolved over the decades. In 1995, Stuart Russell and Peter Norvig defined AI based on rationality and thinking. They came up with two approaches: human and ideal. Under the human approach, they describe AI as systems that think and act like humans. Under the ideal approach, they describe AI as systems that think and act rationally. In 2004, John McCarthy described AI as intelligent machines emulating human intelligence. He noted AI was capable of even exceeding human intelligence.
At its core, AI combines computer science with data science to emulate human cognitive functions. It leverages advanced algorithms and data processing capabilities to operate through a diverse range of personal and professional applications. Examples of AI found in our daily lives include:
Assistants Like Siri, Alexa, and Google Assistant. These AI-powered assistants help people with their daily activities, such as scheduling a meeting or finding the best route to a destination.
Financial AI Applications. These apps analyze vast amounts of financial data to predict market trends and help people optimize their investment portfolios.
Online Shopping. AI programs on retail sites suggest products shoppers may like based on their preferences.
Social Media: Social media algorithms personalize users’ content feeds according to their interests.
Smart Home Devices: These AI-powered devices can control the lights, thermostats, and appliances in a home.
Types of AI
There are various types of AI, each with a different purpose and use. Weak AI (or narrow AI) is a type of AI that excels in specific domains but lacks general humanlike intelligence. A chatbot is an example of weak AI. Natural language processing (NLP) is a type of AI that enables machines to understand, interpret, and generate human language. For example, ChatGPT generates content based on existing data and user prompts. Other AI types in use today include:
Deep Learning: A subset of machine learning, deep learning uses neural networks to process and learn from complex data.
Generative AI: Another subset of AI, generative AI can create new content (text, images, audio, etc.) based on learned data.
Computer Vision: These AI systems enable image recognition, object detection, and facial recognition.
Robotic Process Automation (RPA): These software robots automate and improve efficiency in business processes.
AI technology is constantly evolving, and new types of AI will emerge in the future. Types of AI that are still in development include:
Artificial General Intelligence (AGI): Also called strong AI, AGI includes machines that think and learn like humans and work well across many domains.
Quantum AI: This AI concept combines with quantum computing to tackle complex problems faster and more efficiently.
What Is Machine Learning?
Machine learning is a type of artificial intelligence that enables software to make predictions. It uses only historical data, eliminating the need for programming. The four primary training models are supervised, unsupervised, semi-supervised, and reinforcement learning. Choosing which one to use hinges on the data type a data scientist or analyst wants to use and the desired outcome.
Supervised Learning
Supervised learning uses labeled or structured data to classify data and predict outcomes. Supervised learning algorithms cross-validate new data to ensure it fits the model. A use case for supervised learning is predictive analytics. Using historical data, it can forecast future events, such as customers’ behavior or stock price changes.
Unsupervised Learning
Unsupervised learning can also make predictions using data. The difference is that unsupervised learning can use unlabeled datasets. An unsupervised learning algorithm can autonomously identify patterns and connections between dataset variables. Unsupervised learning can still derive insights when no labels exist within the data. This method can be helpful in product development. For example, unsupervised learning can identify target markets for new products.
Semi-supervised Learning
Semi-supervised learning mixes different types of data to teach predictive models. For example, it can mix small amounts of labeled data with larger amounts of unlabeled data. This method is useful for tasks such as web content classification. While labeling the vast volume of web content available is virtually impossible, semi-supervised learning can use labeled and unlabeled data to quickly categorize content and help improve search results.
Reinforcement Learning
Reinforcement learning trains models to optimize their decisions based on their interactions, and trial and error. It works based on a rewards model. This means the model is rewarded for desired behaviors to reinforce its learning. Reinforcement learning is useful for customer support. For example, a chatbot can refine its responses based on its interactions with users.
Machine Learning vs. AI: The Key Similarities
Machine learning and AI are often mistakenly considered to be the same thing. A key reason is that they both help create intelligent machines. These machines are capable of tasks that demand human intelligence.
A comparison of AI vs. machine learning reveals another key similarity: data. Each relies on data that is used for analysis, to draw conclusions, and to make predictions. For example, predictions made by machine learning use data extracted and analyzed by an AI algorithm.
Machine learning and AI are also similar in purpose. For example, data scientists use them to automate tasks and solve complex problems. In the business realm, both machine learning and AI can ease decision-making. For example, they can help find patterns in data and present them in a way that is easier to understand and use to take action.
Considering a career in AI & Machine Learning?
Learn more about the University of San Diego part-time AI & Machine Learning bootcamp.
Machine Learning vs. AI: The Key Differences
When comparing machine learning vs. AI, it’s important to note that AI is a broader term, encompassing not only machine learning but also other types of AI such as generative AI and computer vision. AI can also include certain techniques, like rule-based systems, expert systems, and knowledge representation.
As a subset of AI, machine learning applies AI’s automation capabilities to data-driven processes and concepts. It is used to train algorithms to learn patterns from data. It then allows other types of AI to make predictions or decisions based on what it has learned.
As for AI and machine learning career pathways, professionals can pursue a number of different roles:
Business Analyst. A business analyst or data analyst can use Python—an essential language used in machine learning—to help translate the value uncovered in data into insights that can be used to make business decisions.
Data Scientist. A data scientist uses knowledge of core data science processes and skills in data wrangling, data exploration, and hypothesis building and testing.
Machine Learning Engineer. A machine learning engineer needs to understand machine learning models and accuracy metrics, deep learning concepts, and deep neural networks.
Prepare for the Future Today
AI and machine learning innovations are becoming integral parts of our daily lives. With the help of these technologies, for instance, voice assistants can help people answer questions, and doctors can uncover ways to improve patients’ lives.
In turn, a favorable shift has occurred in AI and machine learning employment trends. The U.S. Bureau of Labor Statistics projects that the employment of data scientists will grow 36% from 2021 to 2031. This is much faster than the average growth projected for all occupations.
Individuals looking to enhance their knowledge and skills in the field and learn more about the contrasts in machine learning vs. AI can enroll in University of San Diego’s live online AI and Machine Learning Bootcamp. The program teaches in-demand skills using tools such as Python, Keras, and TensorFlow. It also covers advanced concepts ranging from data wrangling to deep neural networks.
Learn how University of San Diego’s AI and Machine Learning Bootcamp can help you pursue your professional goals in the AI field of today and tomorrow.
Recommended Readings
Data Analytics for Beginners: Tips for Getting Started
What Is Product Design? Definition and Overview
What Is Penetration Testing? Skills and Uses
Sources:
Dataiku, “Supervised vs. Unsupervised Machine Learning: Use Cases and Examples”
Forbes, “How Machine Learning Will Transform Your Industry”
Forbes, “What Jobs Are Available in Artificial Intelligence? How to Make a Career in This AI Boom”
Harvard Business Review, “Using AI to Track How Customers Feel—in Real Time”
IBM, “What Is Artificial Intelligence?”
IBM, “What Is Supervised Learning?”
Indeed, 12 Machine Learning and Artificial Intelligence Jobs
Machine Learning Pro, “Semi-supervised Learning: Examples, Benefits and Limitations”
McKinsey & Company, “The State of AI in 2022—and a Half Decade in Review”
Spherical Insights, “Global Machine Learning Market Size to Reach Around USD 302.62 Billion by 2030”
Statista, Artificial Intelligence (AI) Market Size Worldwide in 2021 With a Forecast Until 2030
TechTarget, “Machine Learning”
ZDNet, “What Is AI? Everything to Know About Artificial Intelligence”